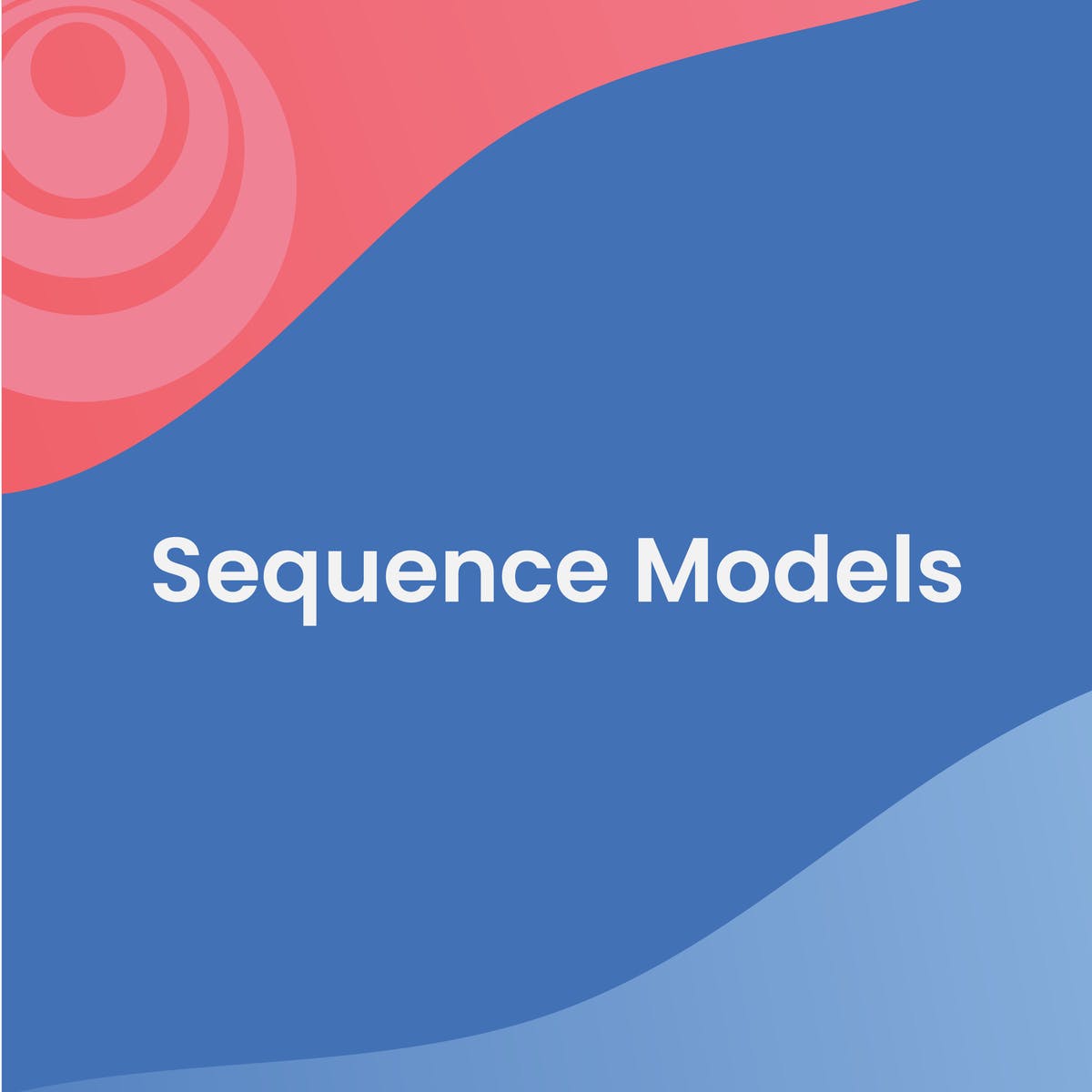
Researchers should explore different approaches to making AI more accessible. Some explanation methods explain why AI makes decisions. Others provide an explanation that doesn't depend on the context. Hence, they may provide a wildly implausible explanation. Some people try to use knowledge-based systems to explain things more in context. No matter which approach you choose to take, it is important that you understand the context.
Explanations should be interactive
Designing an interactive, beneficial system of artificial intelligence is the first step in creating an explainable system. This is because people's preferences, past experiences and choices can impact their decisions. It is important for system owners to remember that people often interpret similar explanations in a different way. An explanation should be interactive to show the system's adaptability and customization to each user.
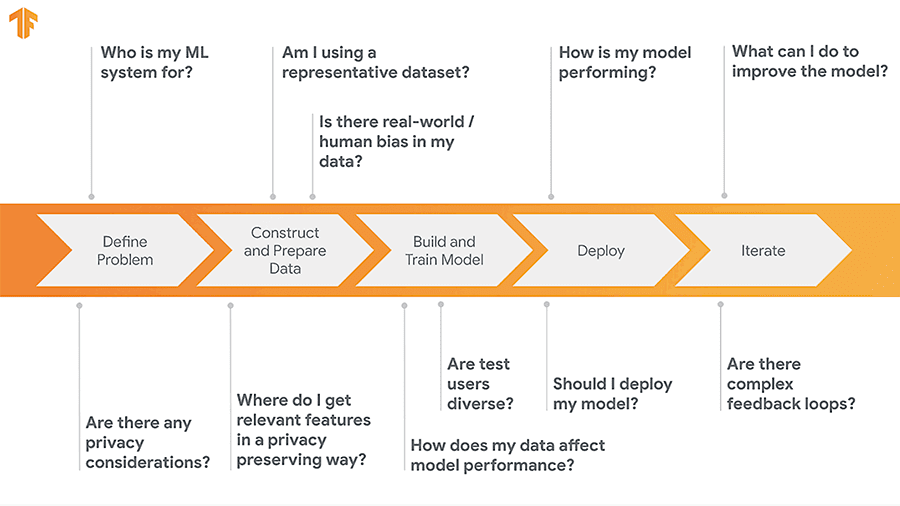
A second step to creating an explicable artificial intelligence application is to think about the level of detail users require. A counterfactual explanation can be enough to explain the smallest change in the model's features, while an interactive explanation will require more work. Contrary to the counterfactual explanation, it will only describe the output of a system and not reveal its inner workings. This explanation can also be used to protect intellectual property.
Interactive AI systems must be able to combine diverse data in order to yield a relevant result. An AI system that can't provide such detailed explanations isn't suitable for clinical usage. It is also necessary that human experts can understand and interpret machine decision-making. This requires trusting the machine's decisions and a high degree of confidence. Personalized medicine requires a high degree of explanationability.
Background knowledge should be used to provide meaningful semantics
We will be discussing how background knowledge can help provide meaningful semantics to explainable AI systems. Background knowledge can also be acquired through domain knowledge. Experiments are another way to acquire background knowledge. Background knowledge is useful for explaining human-machine interactions. In order to increase performance, background knowledge may be added back into a subsymbolic model.
Psychology has long recognized the importance of background knowledge in explaining why things work. Research has shown that explanations are socially-oriented, and include semantic information. It is crucial for effective knowledge transmission. Hilton (1990) explains that explanations can be understood as social interactions and semantic data. Kulesza et al. (2013) also found a positive association between mental models as well as explanation properties. The authors also observed a relationship of completeness with soundness and trust.
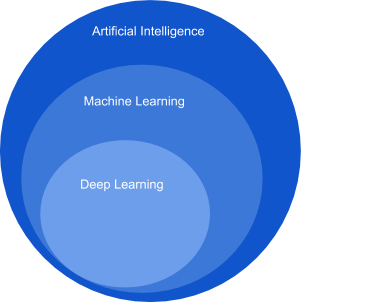
As the use of AI increases, so do the demands for explainability. It is essential to have explanations that are transparent and trustworthy for AI systems. Understanding the user levels is critical to create explainable artificial intelligent systems that can win public trust. This will enable AI systems to trust humans. To understand how AI systems work, you should consider the following background information.
FAQ
What uses is AI today?
Artificial intelligence (AI), a general term, refers to machine learning, natural languages processing, robots, neural networks and expert systems. It's also known as smart machines.
Alan Turing, in 1950, wrote the first computer programming programs. He was interested in whether computers could think. In his paper, Computing Machinery and Intelligence, he suggested a test for artificial Intelligence. The test tests whether a computer program can have a conversation with an actual human.
John McCarthy introduced artificial intelligence in 1956 and created the term "artificial Intelligence" through his article "Artificial Intelligence".
Today we have many different types of AI-based technologies. Some are easy and simple to use while others can be more difficult to implement. They range from voice recognition software to self-driving cars.
There are two major categories of AI: rule based and statistical. Rule-based uses logic for making decisions. To calculate a bank account balance, one could use rules such that if there are $10 or more, withdraw $5, and if not, deposit $1. Statistics is the use of statistics to make decisions. For example, a weather prediction might use historical data in order to predict what the next step will be.
Is Alexa an AI?
Yes. But not quite yet.
Amazon's Alexa voice service is cloud-based. It allows users interact with devices by speaking.
The Echo smart speaker was the first to release Alexa's technology. However, similar technologies have been used by other companies to create their own version of Alexa.
Some examples include Google Home (Apple's Siri), and Microsoft's Cortana.
Why is AI so important?
In 30 years, there will be trillions of connected devices to the internet. These devices will include everything from fridges and cars. Internet of Things, or IoT, is the amalgamation of billions of devices together with the internet. IoT devices will communicate with each other and share information. They will also make decisions for themselves. A fridge may decide to order more milk depending on past consumption patterns.
It is predicted that by 2025 there will be 50 billion IoT devices. This represents a huge opportunity for businesses. It also raises concerns about privacy and security.
What do you think AI will do for your job?
AI will eventually eliminate certain jobs. This includes jobs such as truck drivers, taxi drivers, cashiers, fast food workers, and even factory workers.
AI will create new employment. This includes data scientists, project managers, data analysts, product designers, marketing specialists, and business analysts.
AI will simplify current jobs. This includes positions such as accountants and lawyers.
AI will make it easier to do the same job. This includes jobs like salespeople, customer support representatives, and call center, agents.
Statistics
- More than 70 percent of users claim they book trips on their phones, review travel tips, and research local landmarks and restaurants. (builtin.com)
- That's as many of us that have been in that AI space would say, it's about 70 or 80 percent of the work. (finra.org)
- By using BrainBox AI, commercial buildings can reduce total energy costs by 25% and improves occupant comfort by 60%. (analyticsinsight.net)
- In 2019, AI adoption among large companies increased by 47% compared to 2018, according to the latest Artificial IntelligenceIndex report. (marsner.com)
- While all of it is still what seems like a far way off, the future of this technology presents a Catch-22, able to solve the world's problems and likely to power all the A.I. systems on earth, but also incredibly dangerous in the wrong hands. (forbes.com)
External Links
How To
How do I start using AI?
One way to use artificial intelligence is by creating an algorithm that learns from its mistakes. This allows you to learn from your mistakes and improve your future decisions.
If you want to add a feature where it suggests words that will complete a sentence, this could be done, for instance, when you write a text message. It would take information from your previous messages and suggest similar phrases to you.
It would be necessary to train the system before it can write anything.
Chatbots are also available to answer questions. If you ask the bot, "What hour does my flight depart?" The bot will respond, "The next one departs at 8 AM."
Take a look at this guide to learn how to start machine learning.